自然语言处理中的Attention机制总结
       在面试的过程中被问到了attention,原来虽然其实已经实际用过attention了,也
在面试的过程中被问到了attention,原来虽然其实已经实际用过attention了,也知道个大概原理是加权求和,但是对于加权的具体方法以及权值得分的计算并不是很清晰,面试答的一般,正好最近实习的地方要讲attention机制,所以就跟着多学习了一下,在此做一个总结。
因为这篇文章基本上是参考了很多前人的总结性文章,所以我先把参考的文章放到前面,觉得写得不是很清楚的地方的可以直接去看我参考的文章来看,
参考文章
本篇文章讲解的整体结构
- seq2seq中的简单attention机制
- attention机制的通用定义(Stanford课件中的定义)
- attention的各种变体
- 更多attention种类
- 示例代码
- 总结
下面就开始正式开始博客内容。
1. seq2seq中的attention机制
首先我们快速过一下seq2seq中的attention机制,要了解seq2seq模型的话,不妨去参考上面的雷锋网那篇文章,这里我就快速过一下。下面的图都是seq2seq的示意图,先放的图,然后再放的图片描述:
- 我们在encoder的过程中保留每个RNN单元的隐藏状态(hidden state)得到(h1……hN)
- 然后对于decoder的每一个timestep,因为有此时decoder的输入和上一步的隐藏状态输出,所以我们可以得到当前步的隐藏状态。假设第t步的(根据上一步隐藏状态输出与当前输入得到的)隐藏状态为St,
在每个第t步利用St和hi进行dot点积得到attention score,也称为“相似度“或者“影响度”,或者“匹配得分”
PS.(注意,看到多个网友提出这里的attention score应该用st-1和hi来算。其实最开始的论文在encoder-decoder里面的当前decoder的attention得分用的是st-1和hi来算,但斯坦福教材上图上确实是画的st和hi来算,而且后续论文大多是用的这种方式,即当前步的attention score用的当前步的隐藏状态st和前面的hi去算的,感兴趣的可以看下面的论文,自己理解吧,对应的TensorFlow.contrib.seq2seq里面的两种attention机制。
原始论文:https://arxiv.org/pdf/1409.0473.pdf 对应tf.contrib.seq2seq.BahdanauAttention 计算方式St-1->at->ct->St
后面论文:https://arxiv.org/pdf/1508.04025.pdf 对应tf.contrib.seq2seq.LuongAttention 计算方式(St-1,input)->St->at->ct->S’t
我觉得这种细节性的地方,因为我参考的是斯坦福的教程,所以后面都统一的attention score是使用st和hi来的,大家仁者见仁智者见智,不用喷的那么厉害,我是个彩笔我承认好吧,大家合理讨论就好)
α t = s o f t m a x ( e t ) {{\alpha }^{t}}=softmax ({{e}^{t}}) αt=softmax(et)
利用softmax函数将attention scores转化为概率分布。
a t = ∑ i N α i h i {{\mathrm{a}}_{\mathrm{t}}}=\sum\limits_{i}^{N}{{{\alpha }_{i}}}{{\mathrm{h}}_{\mathrm{i}}} at=i∑Nαihi
按照刚才的概率分布,计算encoder的hidden states的加权求和。到这里其实attention的计算就结束了,得到的这个at就已经是decoder的第t时刻的注意力向量了(在后面的文章中,这个 a t \mathrm{a}_{t} at也称作是上下文向量,context vector,符号表示也可能是用 c t c_{t} ct来表示的)。
最后将注意力向量at,以及decoder的t时刻的hidden state st,并联起来,然后做后续的步骤(比如加个dense全连接层做标签预测之类的。
到这里,seq2seq的最简单最基础的attention机制,就讲完了,如果还有疑问的同学,可以去看原始论文
2. attention的一个通用定义
按照Stanford大学课件上的描述,attention的通用定义如下:
- 给定一组向量集合values,以及一个向量query,attention机制是一种根据该query计算values的加权求和的机制。
- attention的重点就是这个集合values中的每个value的“权值”的计算方法。
- 有时候也把这种attention的机制叫做query的输出关注了(或者说叫考虑到了)原文的不同部分。(Query attends to the values)
举例:刚才seq2seq中,哪个是query,哪个是values?
each decoder hidden state attends to the encoder hidden states (decoder的第t步的hidden state----st是query,encoder的hidden states是values)
从定义来看Attention的感性认识:
- The weighted sum is a selective summary of the information contained in the values, where the query determines which values to focus on.
换句话说,attention机制就是一种根据某些规则或者某些额外信息(query)从向量表达集合(values)中抽取特定的向量进行加权组合(attention)的方法。简单来讲,只要我们从部分向量里面搞了加权求和,那就算用了attention。
3. attention的计算变体
首先从大的概念来讲,针对attention的变体主要有两种方式:
1.一种是在attention 向量的加权求和计算方式上进行创新
2.另一种是在attention score(匹配度或者叫权值)的计算方式上进行创新
当然还有一种就是把二者都有改变的结合性创新,或者是迁移性创新,比如借鉴CNN的Inception思想等等,后续会提到一点,详细的应该是在以后可能要讲的Tranformer里面会详细提到。
我们先针对第一种方法讲讲区别,其实虽然名字变来变去,他们的差异没有那么多。
3.1 针对attention向量计算方式变体
大概分成这么几种:
-
Soft attention、global attention、动态attention
-
Hard attention
-
“半软半硬”的attention (local attention)
-
静态attention
-
强制前向attention
Soft attention、global attention、动态attention
这三个其实就是Soft attention,也就是我们上面讲过的那种最常见的attention,是在求注意力分配概率分布的时候,对于输入句子X中任意一个单词都给出个概率,是个概率分布,把attention变量(context vecor)用 c t c_t ct表示,attention得分在经过了softmax过后的权值用 a l p h a alpha alpha表示
论文:Neural machine translation by jointly learning to align and translate
Hard attention
Soft是给每个单词都赋予一个单词match概率,那么如果不这样做,直接从输入句子里面找到某个特定的单词,然后把目标句子单词和这个单词对齐,而其它输入句子中的单词硬性地认为对齐概率为0,这就是Hard Attention Model的思想。
Hard attention的这个pt,我也没有详细研究,不过我觉得可能跟下面讲的local attention机制的找法差不多。据说Hard attention 一般用在图像里面,当图像区域被选中时,权重为1,剩下时候为0。
https://www.cnblogs.com/Determined22/p/6914926.html
local attention (半软半硬attention)
Soft attention 每次对齐的时候都要考虑前面的encoder的所有hi,所以计算量会很大,因此一种朴素的思想是只考虑部分窗口内的encoder隐藏输出,其余部分为0,在窗口内使用softmax的方式转换为概率。这个local attention相反概念的是global attention,global attention其实就是softmax attention,这里不多赘述global attention了。
论文:Effective Approaches to Attention-based Neural Machine Translation
在这个模型中,对于是时刻t的每一个目标词汇,模型首先产生一个对齐的位置 pt(aligned position),context vector 由编码器中一个集合的隐藏层状态计算得到,编码器中的隐藏层包含在窗口[pt-D,pt+D]中,D的大小通过经验选择。
上式之中,大S指的是源句子的长度,Wp和vp是指的模型的参数,通过训练得到,为了支持pt附近的对齐点,设置一个围绕pt的高斯分布,其中小s是在以pt为中心的窗口中的整数,pt是一个在[0,S]之间的实数。小Sigma σ 一般取窗口大小的一半。
静态attention
静态attention:对输出句子共用一个St的attention就够了,一般用在Bilstm的首位hidden state输出拼接起来作为st(在图所示中为u)
论文:Teaching Machines to Read and Comprehend 以及
Supervised Sequence Labelling with Recurrent Neural Networks
上面这个图是我从论文摘过来的静态attention的示意图,有同学可能会注意到的是:这个前面的每个hidden state 不应该都和这里的 u 算一次attention score吗,怎么这里只有一个r和u进行了交互?
其实他这里的r表示的是加权平均的self attention,这个权就是attention ct向量,这个图里面把attention ct的计算过程省略了。直接跳到了ct和st计算真正的s’t的部分。他这个里面用的实际的attention score的计算并不是用点积,是additive attention,什么是additive attention呢?这个下面就会讲根据按照attention score计算的不同的attention model变体。
3.2 针对Attention score的计算方式变体
讲完了在加权向量 α i \alpha_i αi(注意,此处这个 α i \alpha_i αi指的是得分softmax之后的向量的第i个分量)的计算上面的各种attention变体,我们现在再回到原始的soft attention公式上面来。在softmax 的Attention向量的计算总是依赖于权重求和,而权重往往是attention score的softmax。
我们可以看到,式子中的变量e代表的就是attention score,alpha是attention的权重,a就是context vector
根据attention score的计算方式不同,我们可以将attention进一步细分分类。attention score的计算主要有以下几种:
注意点积attention score这里有个假设,就是s和h的维数要一样才能进行点积,很好理解。
第二个W矩阵是训练得到的参数,维度是d2 x d1,d2是s的hidden state输出维数,d1是hi的hidden state维数
最后就是上面提到的additive attention,是对两种hidden state 分别再训练矩阵然后激活过后再乘以一个参数向量变成一个得分。
其中,W1 = d3xd1,W2 = d3*d2,v = d3x1 ,d1,d2,d3分别为h和s还有v的维数,属于超参数。
4.更加特殊的attention
4.1 self attention
- 思想:Self attention也叫做intra-attention在没有任何额外信息的情况下,我们仍然可以通过允许句子使用–self attention机制来处理自己,从句子中提取关注信息。
- 它在很多任务上都有十分出色的表现,比如阅读理解 (Cheng et al., 2016) 、文本继承 (textual entailment/Parikh et al., 2016) 、自动文本摘要 (Paulus et al., 2017) 。
其已经被证明是非常有效的,而且所以我们下面重点关注一下。
论文:
Cheng, J., Dong, L., & Lapata, M. (2016). Long Short-Term Memory-Networks for Machine Reading
Parikh, A. P., Täckström, O., Das, D., & Uszkoreit, J. (2016). A Decomposable Attention Model for Natural Language Inference. In Proceedings of the 2016 Conference on Empirical Methods in Natural Language Processing.
Paulus, R., Xiong, C., & Socher, R. (2017). A Deep Reinforced Model for Abstractive Summarization. In arXiv preprint arXiv:1705.04304,.
Self attention计算方式
- 以当前的隐藏状态去计算和前面的隐藏状态的得分,作为当前隐藏单元的attention score,例如:
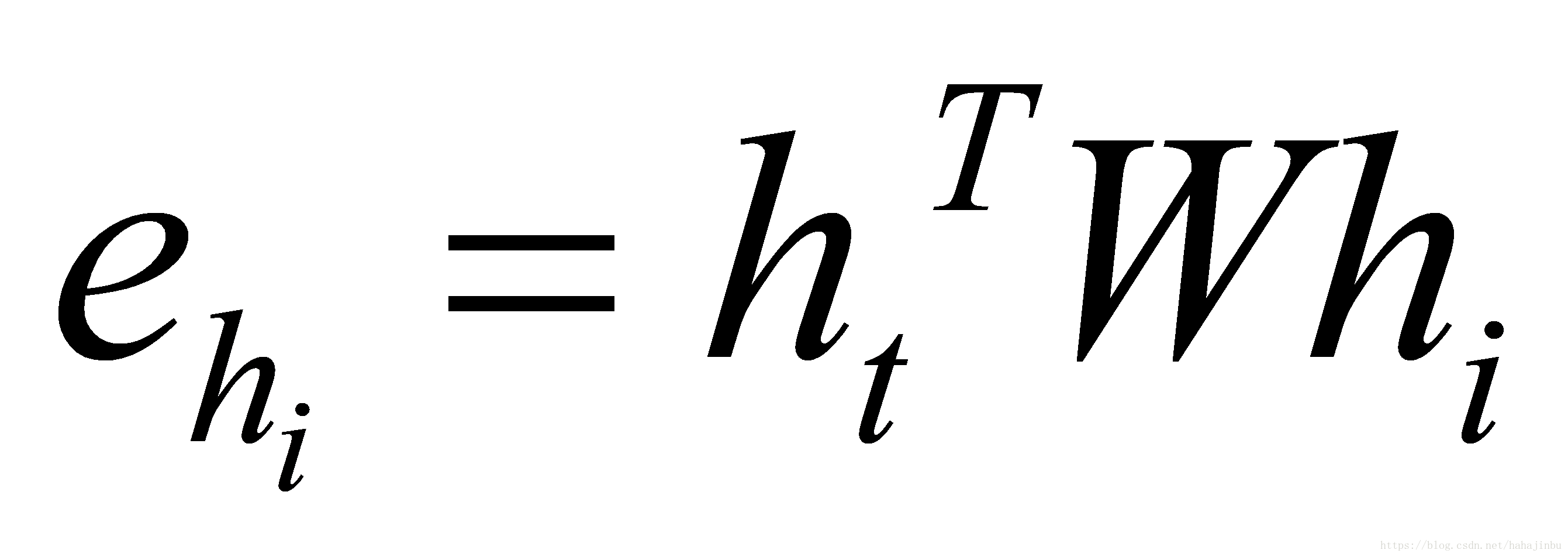
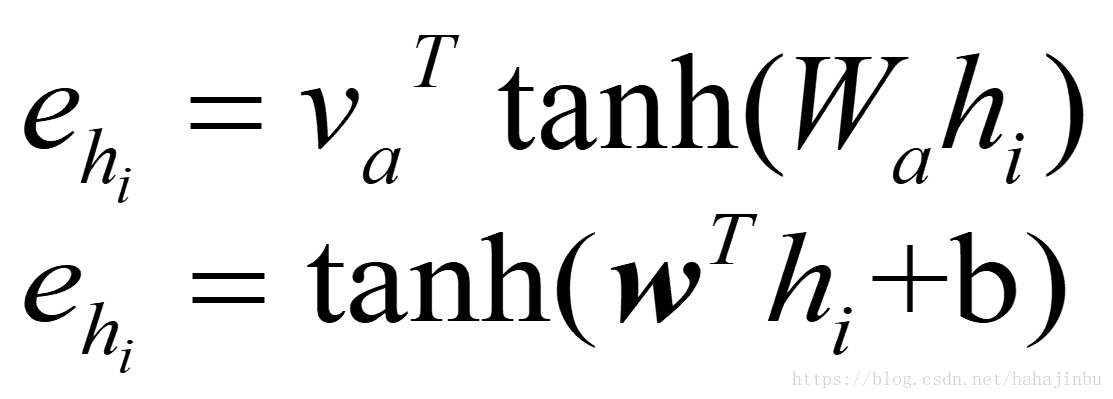
其中va和Wa是参数,通过训练得到,Wa维数dxd,hi维数dx1,va维数dx1(第一个公式)
前面的w是dx1的向量,hi是dx1,b是(1,)(第二个公式),注意这里其实是简化过后的表示:原始论文 https://arxiv.org/pdf/1512.08756.pdf
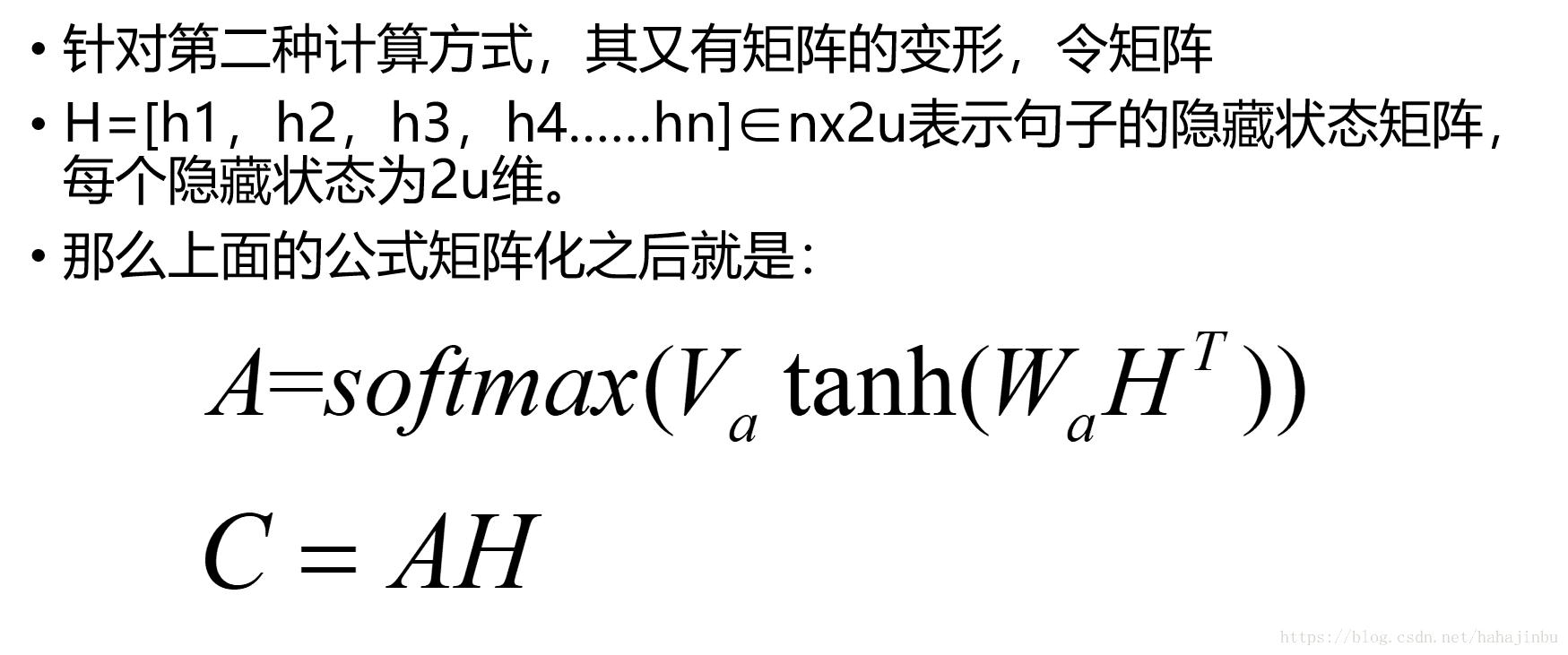
上面的式子中,使用的va向量是一个通用的向量,每个隐藏状态并没有区分,如果我们对不同状态计算的时候学习不同的向量va,,也就是一个Va矩阵,得到的就是一个attention矩阵。
论文:
https://arxiv.org/pdf/1703.03130.pdf
上面的式子中,H是nx2u(双向lstm的结果拼接,每个单向LSTM的hidden units是u),Wa是dx2u,Va是rxd,得到的attention 矩阵 A是rxn。
C = rx2u,按论文意思来看就是把一句话编码成了一个rx2u的矩阵(sentence embedding)
在实际操作的过程中,我们也可能在学习时候给A一个F2范式约束项来避免过拟合。
key-value attention
这种attention就是比较新的了,简单来说Key-value attention 是将hi拆分成了两部分[ k e y t key_t keyt; v a l u e t value_t valuet],然后使用的时候只针对key部分计算attention权重,然后加权求和的时候只使用value部分进行加权求和。公式如下,attention权重计算如下:
论文:Daniluk, M., Rockt, T., Welbl, J., & Riedel, S. (2017). Frustratingly Short Attention Spans in Neural Language Modeling. In ICLR 2017.
其中呢,这个L是attention窗口长度
WY=kxk,ki=kx1,Wh = kxk,1^T是一个1xL的向量
w 是一个kx1的向量
Wr = kxk,
Wx = kxk
最后得到kx1的ht*与ht一起参与下一步的运算
4.2 multi-head attention
最后简单讲一下google的attention is all you need 里面的attention,这一段基本上是摘抄的苏剑林的科学空间的博文了。
Google 在 attention is all you need 中发明了一种叫transformer的网络结构,其中用到了multi-head attention。Transformer在下一节课里面会讲,所以我们就简单介绍一下他里面用到的attention。
首先,google先定义了一下attention的计算,也是定义出key,value,query三个元素(在seq2seq里面,query是st,key和value都是hi)在self 里面,query 是当前要计算的hi,k和v仍然一样,是其他单元的hidden state。在key value attention里面key和value则是分开了的。
然后除以了一下根号dk,为了让内积不至于太大(太大的话softmax后就非0即1了,不够“soft”了)
这里我们不妨假设,Q是nxdk,K是mxdk,v是mxdv,忽略归一化和softmax的话就是三个矩阵相乘,得到的是n*dv的矩阵。我们可以说,通过这么一个attention层,就将一个nxdk的序列Q,提取信息编码成nxdv的序列了。
Wi用来先在算attention对三个矩阵做不同的矩阵变换映射一下,变成nxdk’,mxdk’,mxdv’维度。
最后做并联,有点类似于inception 里面多个卷积核的feature map并联的感觉。
4.3 channel attention
CV里面呢,有一种新的attention机制流行了起来,最出名的文章是Squeeze-and-excitation networks,里面就是这个模块就是先将一个whc的图像,做globalpooling之后压缩再还原作为权重乘上原来的feature map。
Hu J, Shen L, Sun G. Squeeze-and-excitation networks[C]//Proceedings of the IEEE conference on computer vision and pattern recognition
那么,如果我们想要在NLP里面中,用上channel attention,应该怎么用呢?
笔者认为,可以这样做一个简单的实现并迁移:
### keras 代码
time_step = 512
emb_size = 128
input = Input(shape=(time_step,emb_size)) #
g_pooling = Lambda(lambda x : K.mean(x,axis=-1))(input) #bs,time_step
squeeze = Dense(time_step // 4, activation='relu')(g_pooling) #bs,512//4
scale = Dense(time_step,activation='sigmoid')(squeeze) #bs,time_step
scale = Lambda(lambda x : x[...,tf.newaxis])(scale) #bs,time_step,1
attention_feature = multiply([input,scale])
这个思路就是我们将一句话的中的每个词的词向量(1emb_size)当做cnn里面的wh,然后每个timestep当做channel,这样做了把每个词的average pooling之后当做这个词的表征来算一下attention,这种设计笔者个人认为在一些很长的句子的时候,可能会有一点用(比如举例的timestep是512个词)
另一种实现思路是同样把句子真的过几个textCNN变成多channel的,这样的话,每个channel就代表了整个句子的表征,哪种好的话可以尝试一下,我自己是没试过的:
time_step = 512
emb_size = 128
input = Input(shape=(time_step,emb_size)) #
conv = Conv1D(32 ,3,padding='same')(input) #bs,time_step,filter_size ##这里也可以多来几个,concat
g_pooling = Lambda(lambda x : K.mean(x,axis=1,keepdims=True))(conv) #bs,1,filter_size
filter_num = K.int_shape(g_pooling)[-1]
squeeze = Dense(filter_num// 4, activation='relu')(g_pooling) #bs,1,filter_size//4
scale = Dense(filter_num,activation='sigmoid')(squeeze) #bs,1,filter_size
attention_feature = multiply([conv,scale])
5. 代码示例
以在网上找到的一段keras实现的self attention的代码,其实现原理应该是这篇论文,结构如上图所示,是利用一个mlp来计算h(t)的attention得分,然后这个代码里面进行了进一步简化,让把mlp简化得只有一个共享的w向量,但是每个ht有一个不同的偏置。
类似于 α ( h t ) = t a n h ( w T h t + b t ) \alpha(h_t)=tanh(w^T{h_t}+b_t) α(ht)=tanh(wTht+bt)的感觉。
#coding=utf-8
'''
Single model may achieve LB scores at around 0.043
Don't need to be an expert of feature engineering
All you need is a GPU!!!!!!!
The code is tested on Keras 2.0.0 using Theano backend, and Python 3.5
referrence Code:https://www.kaggle.com/lystdo/lstm-with-word2vec-embeddings
'''
########################################
## import packages
########################################
import os
import re
import csv
import codecs
import numpy as np
import pandas as pd
########################################
## set directories and parameters
########################################
from keras import backend as K
from keras.engine.topology import Layer
# from keras import initializations
from keras import initializers, regularizers, constraints
np.random.seed(2018)
class Attention(Layer):
def __init__(self,
W_regularizer=None, b_regularizer=None,
W_constraint=None, b_constraint=None,
bias=True, **kwargs):
"""
Keras Layer that implements an Attention mechanism for temporal data.
Supports Masking.
Follows the work of Raffel et al. [https://arxiv.org/abs/1512.08756]
# Input shape
3D tensor with shape: `(samples, steps, features)`.
# Output shape
2D tensor with shape: `(samples, features)`.
:param kwargs:
"""
self.supports_masking = True
# self.init = initializations.get('glorot_uniform')
self.init = initializers.get('glorot_uniform')
self.W_regularizer = regularizers.get(W_regularizer)
self.b_regularizer = regularizers.get(b_regularizer)
self.W_constraint = constraints.get(W_constraint)
self.b_constraint = constraints.get(b_constraint)
self.bias = bias
self.features_dim = 0
super(Attention, self).__init__(**kwargs)
def build(self, input_shape):
self.step_dim = input_shape[1]
assert len(input_shape) == 3 # batch ,timestep , num_features
print(input_shape)
self.W = self.add_weight((input_shape[-1],), #num_features
initializer=self.init,
name='{}_W'.format(self.name),
regularizer=self.W_regularizer,
constraint=self.W_constraint)
self.features_dim = input_shape[-1]
if self.bias:
self.b = self.add_weight((input_shape[1],),#timesteps
initializer='zero',
name='{}_b'.format(self.name),
regularizer=self.b_regularizer,
constraint=self.b_constraint)
else:
self.b = None
self.built = True
def compute_mask(self, input, input_mask=None):
# do not pass the mask to the next layers
return None
def call(self, x, mask=None):
features_dim = self.features_dim
step_dim = self.step_dim
print(K.reshape(x, (-1, features_dim)))# n, d
print(K.reshape(self.W, (features_dim, 1)))# w= dx1
print(K.dot(K.reshape(x, (-1, features_dim)), K.reshape(self.W, (features_dim, 1))))#nx1
eij = K.reshape(K.dot(K.reshape(x, (-1, features_dim)), K.reshape(self.W, (features_dim, 1))), (-1, step_dim))#batch,step
print(eij)
if self.bias:
eij += self.b
eij = K.tanh(eij)
a = K.exp(eij)
# apply mask after the exp. will be re-normalized next
if mask is not None:
# Cast the mask to floatX to avoid float64 upcasting in theano
a *= K.cast(mask, K.floatx())
a /= K.cast(K.sum(a, axis=1, keepdims=True) + K.epsilon(), K.floatx())
print(a)
a = K.expand_dims(a)
print("expand_dims:")
print(a)
print("x:")
print(x)
weighted_input = x * a
print(weighted_input.shape)
return K.sum(weighted_input, axis=1)
def compute_output_shape(self, input_shape):
# return input_shape[0], input_shape[-1]
return input_shape[0], self.features_dim
6. 总结
- 总的来说,attention的机制就是一个加权求和的机制,只要我们使用了加权求和,不管你是怎么花式加权,花式求和,只要你是根据了已有信息计算的隐藏状态的加权和求和,那么就是使用了attention,而所谓的self attention就是仅仅在句子内部做加权求和(区别与seq2seq里面的decoder对encoder的隐藏状态做的加权求和)。
- self attention我个人认为作用范围更大一点,而key-value其实是对attention进行了一个更广泛的定义罢了,我们前面的attention都可以套上key-value attention,比如很多时候我们是把k和v都当成一样的算来,做self的时候还可能是quey=key=value。
最后,我把分享的ppt也放到文库了,免积分下载(设置未成功,默认要1积分…),有需要的人自取吧。
大佬同学自己搞了个公众号,帮忙宣传一波,wx公众号“包包算法笔记”。公众号的主人是我的同学,kaggle的GrandMaster,而且这个大哥为人幽默有趣,心态开放。目前公众号里面主要聊的都是自己的算法见解和经验分享,当然也有自己的一些最近的活动啥的,干货也比较多,最良心的没有卖课广告,不会像各种所谓的技术公众号一样,先给你制造一波忧虑,然后告诉你“买了公众号分享的课之后你就无敌了”一样打广告。
感兴趣的同学不妨关注一下~
更多推荐
所有评论(0)