模式识别和机器学习实战-K近邻算法(KNN)- Python实现 - 约会网站配对效果判断和手写数字识别
文章目录前言一、 k-近邻算法的Python实现1.**工作原理**2.举个简单例子:3.步骤描述来了——代码实现总结前言K-近邻算法(k-Nearest Neighbor algorithm),又称为KNN算法,是数据挖掘技术中最简单的方法一、 k-近邻算法的Python实现1.工作原理存在一个样本的数据集合,也称作训练样本集,样本集中每个数据存在标签,输入没有标签的新数据以后,将新数据的每个特
文章目录
前言
K-近邻算法(k-Nearest Neighbor algorithm),又称为KNN算法,是数据挖掘技术中最简单的方法,属于机器学习实践中的入门,这篇文章主要是用于上机操作。
先介绍和解释步骤,分段给出代码;最后再给出源码,只需修改一下文件路径即可运行(和py文件放在同一目录下)
而具体的文件和代码,在如下链接里:机器学习-KNN算法的python实现 - 数据集和源码
推荐使用Jupyter Notebook ,当然用pycharm也可以。
一、 k-近邻算法(KNN)
1.算法介绍
存在一个样本的数据集合,也称作训练样本集,样本集中每个数据存在标签,输入没有标签的新数据以后,将新数据的每个特征与样本集中的数据特征进行比较,找到与新数据的距离最近的k个“邻居”,如果这k个实例多属于某个类别新数据就属于这个类别。
通常在分类任务中用于投票法,而在回归任务中用平均法,有时候还可以根据距离的远近进行加权,距离越近的权重越大。
简单地说:离X最近的k个点决定X为哪一类
与其他机器学习算法的不同之处在于,KNN是一种 “懒惰学习(lazing learing)” 算法,没有显式的训练过程,仅仅在训练阶段把学习样本保存下来,训练的时间开销为 0,直到测试时才对样本进行处理 (原来机器也会摆烂…)
2.举个例子——电影分类
使用KNN算法 分类一个新的电影是爱情片还是动作片(非真实次数)
打斗镜头和接吻镜头就是电影的特征;电影属于爱情片还是动作片就是标签 (还有爱情动作片是吧)
而度量距离是使用 欧氏距离(二范数)
如图为在二维平面的分类结果,把新的电影归于爱情片
3.步骤描述
k-近邻算法的步骤:
(1)计算已知类别数据集中点与当前点的距离
(2)按照距离递增次序排序
(3)选取与当前点距离最小的k个点
(4)选取当前k个点所在类别的出现频率
(5)返回前k个点出现频率最高的类别作为当前的预测类别
4.来了——代码实现
from numpy import *
import operator
#导入数据
def createData():
group=array([1.0,1.1],[1.0,1.0],[0.0,0.0],[0.0,0.1])
labels=['A','A','B','B']
return group,labels
# KNN分类算法
def classif(inx,dataset,labels,k):
datasetsize=dataset.shape[0]
diffmat=tile(inx,(datasetsize,1))-dataset
DiffMat = diffmat**2
sqDistances = DiffMat.sum(axis=1)
distances=sqDistances**0.5
sortedDistIndicies = distances.argsort()
classcount={}
for i in range(k):
vote=labels[sortedDistIndicies[i]]
classcount[vote]=classcount.get(vote,0)+1
sortedclasscount =sorted(classcount.items(),key=operator.itemgetter(1),reverse=True)
return sortedclasscount[0][0],classcount
# 运行代码
group,labels=createData()
a=classif([0,0],group,labels,3)
运行结果
二、实战之约会网站配对效果判断
1.导入数据
收集约会数据,将这些数据存放在文本文件 datingTestSet2.txt 中,总共1000行,包含三种特征:
每年的飞行里程数,玩游戏视频的时间百分比,每周冰淇淋公升数以及三个标签1,2,3分别表示不喜欢,一般喜欢,很喜欢
(很奇怪,找对象和玩游戏时间有关系我理解,但和飞行多少、吃多少冰激凌有半毛钱关系呀?)
但是这些特征数据想要分类,必须将数据的格式转化为可以接受的格式(例如数组),因此需要有个函数来进行转换
#用于导入数据的函数
def file2matrix(filename):
fr = open(filename)
array0Lines = fr.readlines()
numberOfLines = len(array0Lines)
returnMat = zeros((numberOfLines, 3))
classLabelVector = []
index = 0
for line in array0Lines:
line = line.strip()
listFromLine = line.split('\t')
returnMat[index,:] = listFromLine[0:3]
classLabelVector.append(int(listFromLine[-1]))
index += 1
return returnMat, classLabelVector, array0Lines
#获得数据集
datingDataMat, datingLabels, array0Lines = file2matrix('datingTestSet2.txt')
2.分析数据
可视化最直观,使用matplotlib绘制散点图
import matplotlib.pyplot as plt
fig = plt.figure()
ax = fig.add_subplot(111)
#参数111的意思是:将画布分割成1行1列,图像画在从左到右从上到下的第1块
ax.scatter(datingDataMat[:,1], datingDataMat[:,2], 15.0*array(datingLabels), 15.0*array(datingLabels))
#datingDataMat是上面返回的数组特征,这里1和2分别对应第二列特征和第三列特征
plt.xlabel('Percentage of Time Spent Playing Video Games')#坐标轴名称
plt.ylabel('Liters of Ice Cream Consumed Per Week')
plt.show()
得到结果,如图为“玩游戏视频的时间百分比”,“每周冰淇淋公升数”的分布,不同颜色代表喜好程度;
fig2 = plt.figure()
ax2 = fig2.add_subplot(111)
ax2.scatter(datingDataMat[:,0], datingDataMat[:,1], 15.0*array(datingLabels), 15.0*array(datingLabels))
plt.xlabel('frequent fliters miles per year ')
plt.ylabel('Liters of Ice Cream Consumed Per Week')
plt.show()
得到结果,如图为“每年飞行公里数”,“每周冰淇淋公升数”的分布,不同颜色代表喜好程度;
3.数据归一化
分析图像就会发现,里程数和公斤数这个数据大小相差太多了,那么数据大的将严重影响其它两个特征,那么此时就需要让这三个比值同等重要,此时就需要用到归一化
使用公式:newvalue=(oldvalue-min)/(max-min)
#数据归一化函数,增加一个新的函数autoNorm自动转化到(0,1)区间
def autoNorm(dataSet):
minVals = dataSet.min(0)
maxVals = dataSet.max(0)
ranges = maxVals - minVals
normDataSet = zeros(shape(dataSet))
m = dataSet.shape[0]# 行数
normDataSet = dataSet - tile(minVals, (m,1))
normDataSet = normDataSet/tile(ranges, (m,1)) #element wise divide
return normDataSet, ranges, minVals
# 得到归一化的样本集
normMat, ranges, minVals = autoNorm(datingDataMat)
得到的数据集normMat如图:
4. 测试算法→使用错误率来检测性能
def datingClassTest():
hoRatio = 0.1 #测试集和训练集的比例,测试集和训练集的比例1:9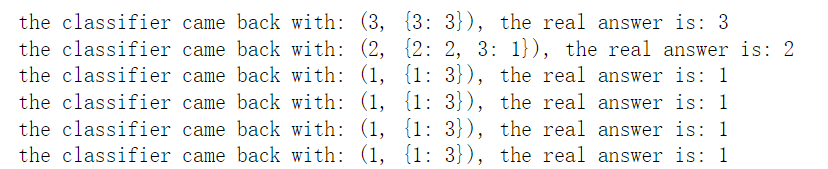
datingDataMat,datingLabels, array0Lines = file2matrix('datingTestSet2.txt')
normMat, ranges, minVals = autoNorm(datingDataMat)
m = normMat.shape[0] # 行数,也是样本总数 m
numTestVecs = int(m*hoRatio)# 取所有样本中的一部分当成测试集
errorCount = 0.0
for i in range(numTestVecs):
classifierResult = classify0(normMat[i,:],normMat[numTestVecs:m,:],datingLabels[numTestVecs:m],3)
print("the classifier came back with: %s, the real answer is: %s" % (classifierResult, datingLabels[i]))
if (classifierResult[0]!= datingLabels[i]): errorCount += 1.0
print("the total error rate is: %f" % (errorCount/float(numTestVecs)))
print(errorCount)
print(numTestVecs)
#运行代码
datingClassTest()
得到的部分结果:
5. 构建完整的系统
此时我们通过输入他人信息来判断海伦对对方的喜欢程度
# 新的样本判断喜欢程度
def classifyPerson():
resultList = ['unlike', 'in small doses', 'in large doses']
percentTats = float(input("percentage of time spent playing video games>"))
ffMiles = float(input("frequent fliters miles per year?"))
iceCream = float(input('liters of ice cream consumed per year?'))
inArr = array([ffMiles, percentTats, iceCream])
classifierResult = classify0((inArr-minVals)/ranges, normMat, datingLabels,3)
print(classifierResult)
print("you will probably like this person:", resultList[classifierResult[0]-1])
#运行代码
classifyPerson()
测试结果如下:
6.总结分析
- 分类时只设定了k=3,没有讨论其他的k值大小;是否选择其他的k值,会对准确率有所提高?
- 测试集和训练集的划分,只是简单的按比例取前10%的数据作为测试集,剩下的为训练集,可以进一步使用交叉验证等方法。
完整的代码:
# 准备数据
def file2matrix(filename):
fr = open(filename)
array0Lines = fr.readlines()
numberOfLines = len(array0Lines)
returnMat = zeros((numberOfLines, 3))
classLabelVector = []
index = 0
for line in array0Lines:
line = line.strip()
listFromLine = line.split('\t')
returnMat[index,:] = listFromLine[0:3]
classLabelVector.append(int(listFromLine[-1]))
index += 1
return returnMat, classLabelVector, array0Lines
# KNN分类算法
def classify0(inX, dataSet, labels, k):
dataSetSize = dataSet.shape[0] # 查看当前数据集有多少列,返回列数
diffMat = tile(inX,(dataSetSize,1)) - dataSet # inX 是待输入数组,(,)使用维度
sqDiffMat = diffMat**2 # 计算两点间距离公式用二次方
sqDistances = sqDiffMat.sum(axis=1)
distances = sqDistances**0.5 # 所有平方和最后根号也就是0.5次方
sortedDistIndicies = distances.argsort() # 排序
classCount={}
for i in range(k): # for循环,前k次
voteIlabel = labels[sortedDistIndicies[i]]
classCount[voteIlabel] = classCount.get(voteIlabel,0)+1
sortedClassCount = sorted(classCount.items(), key=operator.itemgetter(1), reverse=True)
return sortedClassCount[0][0], classCount # 最后返回最高频率元素
#数据归一化
def autoNorm(dataSet):
minVals = dataSet.min(0)
maxVals = dataSet.max(0)
ranges = maxVals - minVals
normDataSet = zeros(shape(dataSet))
m = dataSet.shape[0]
normDataSet = dataSet - tile(minVals, (m,1))
normDataSet = normDataSet/tile(ranges, (m,1))
return normDataSet, ranges, minVals
#测试算法错误率
def datingClassTest():
hoRatio = 0.1
datingDataMat,datingLabels, array0Lines = file2matrix('datingTestSet2.txt')
normMat, ranges, minVals = autoNorm(datingDataMat)
m = normMat.shape[0]
numTestVecs = int(m*hoRatio)
errorCount = 0.0
for i in range(numTestVecs):
classifierResult = classify0(normMat[i,:],normMat[numTestVecs:m,:],datingLabels[numTestVecs:m],3)
print("the classifier came back with: %s, the real answer is: %s" % (classifierResult, datingLabels[i]))
if (classifierResult[0]!= datingLabels[i]): errorCount += 1.0
print("the total error rate is: %f" % (errorCount/float(numTestVecs)))
print(errorCount)
print(numTestVecs)
datingClassTest()
# 进行新的预测
def classifyPerson():
resultList = ['unlike', 'in small doses', 'in large doses']
percentTats = float(input("percentage of time spent playing video games>"))
ffMiles = float(input("frequent fliters miles per year?"))
iceCream = float(input('liters of ice cream consumed per year?'))
inArr = array([ffMiles, percentTats, iceCream])
classifierResult = classify0((inArr-minVals)/ranges, normMat, datingLabels,3)
print(classifierResult)
print("you will probably like this person:", resultList[classifierResult[0]-1])
classifyPerson()
三、实战之手写数字识别
1.准备数据
实际图像存储在文件夹trainingDigits和testDigits中,我们使用trainingDigits去训练分类器,使用testDigits去进行测试分类效果。
我们需要将这个32*32的二进制图像转换为1 * 1024的向量,那么就需要一个函数去进行转化
#把图片变成向量
def img2vector(filename):
returnVect = zeros((1,1024))
fr = open(filename)
#读取文件的32行32列,将其放在returnVector向量中
for i in range(32):
lineStr = fr.readline()
for j in range(32):
returnVect[0,32*i+j] = int(lineStr[j])
return returnVect
testVector = img2vector('testDigits/0_13.txt')
2.使用算法识别手写数字
如图为trainingDigits中的文件名称,开头第一个数字就为图片的标签
手写数字识别代码如下,导入训练集和测试集,并输出错误率
from os import listdir
def handwritingClassTest():
hwLabels = []
trainingFileList = listdir('trainingDigits') # listdir列出给定目录的文件名
m = len(trainingFileList) # m 是文件个数
trainingMat = zeros((m,1024)) #创建m行1024列的训练集汇总矩阵,zeros代表矩阵的元素都是0
for i in range(m):
fileNameStr = trainingFileList[i] # 矩阵的每一行是一个图像的,对行进行操作,先把每行的文件名给到fileNameStr
fileStr = fileNameStr.split('.')[0]
classNumStr = int(fileStr.split('_')[0]) # 把.和_去掉
hwLabels.append(classNumStr) # append作用是将()内内容加到hwLabels数组中,也就是把标签加入
trainingMat[i,:] = img2vector('trainingDigits/%s' % fileNameStr) #使用转换函数转换为1*1024,如此循环i行,将训练集trainingMat填满
#下面是测试集,导入文件时也同样的操作
testFileList = listdir('testDigits')
errorCount = 0.0
mTest = len(testFileList)
for i in range(mTest):
fileNameStr = testFileList[i]
fileStr = fileNameStr.split('.')[0]
classNumStr = int(fileStr.split('_')[0])
vectorUnderTest = img2vector('testDigits/%s' % fileNameStr)
# 使用之间的classify0分类算法,对测试集进行测试,和真实结果对比,可以算出错误率
classifierResult = classify0(vectorUnderTest, trainingMat, hwLabels, 3)
print("the classifier came back with: %d, the real answer is: %d" % (classifierResult[0], classNumStr))
if (classifierResult[0] != classNumStr): errorCount += 1.0
print("\nthe total number of errors is: %d" % errorCount)
print("\nthe total error rate is: %f" % (errorCount/float(mTest)))
#运行识别数字程序
handwritingClassTest()
得到的运行结果如下:(k=3)
3.改进
- 在不同的k值下的识别错误率比较
k=2时
k=4时
k=5时
考虑加上不同k下的错误率统计图,完整的代码如下:
from os import listdir
#把图片变成向量
def img2vector(filename):
returnVect = zeros((1,1024))
fr = open(filename)
#读取文件的32行32列,将其放在returnVector向量中
for i in range(32):
lineStr = fr.readline()
for j in range(32):
returnVect[0,32*i+j] = int(lineStr[j])
return returnVect
#识别数字,输入正整数k
def handwritingClassTest(k):
hwLabels = []
trainingFileList = listdir('trainingDigits')
m = len(trainingFileList)
trainingMat = zeros((m,1024))
for i in range(m):
fileNameStr = trainingFileList[i]
fileStr = fileNameStr.split('.')[0]
classNumStr = int(fileStr.split('_')[0])
hwLabels.append(classNumStr)
trainingMat[i,:] = img2vector('trainingDigits/%s' % fileNameStr)
testFileList = listdir('testDigits')
errorCount = 0.0
mTest = len(testFileList)
for i in range(mTest):
fileNameStr = testFileList[i]
fileStr = fileNameStr.split('.')[0]
classNumStr = int(fileStr.split('_')[0])
vectorUnderTest = img2vector('testDigits/%s' % fileNameStr)
classifierResult = classify0(vectorUnderTest, trainingMat, hwLabels, k)
'''print("the classifier came back with: %d, the real answer is: %d" % (classifierResult[0], classNumStr))'''
if (classifierResult[0] != classNumStr): errorCount += 1.0
print("\n K=%d ,the total number of errors is: %d" % (k,errorCount))
print("\n K=%d ,the total error rate is: %f" % (k,(errorCount/float(mTest))))
return errorCount/float(mTest)
#绘制不同k下的错误率统计图
y = []
for i in range(1, 10):
y.append(handwritingClassTest(i))
fig = plt.figure()
ax = fig.add_subplot(111)
x = [i for i in range(1, 10)]
plt.bar(x, y, align='center')
plt.xlabel('the value of K', )
plt.ylabel('Error rate')
plt.title('K graph')
plt.show()
结果大致如图所示:
- 还可以增加因距离远近的加权比重,这里感兴趣的小伙伴可以自己尝试一下哦
升华主题
KNN算法并不算难,属于机器学习入门基础,现在我们在学习了这个算法后再次想一想,其核心思想能用于解决其他什么问题呢?
- 著名商业哲学家、成功学创始人吉米·罗恩说过:“把你最常接触的五个人平均起来,就是你自己,而这也可以预测你的未来会如何。”
古语有云:物以类聚,人以群分。燕雀不可能与鸿鹄为伍,想要站在群山之巅的人,亦不会与甘于平庸之辈相处。
我们耳熟能详的孟母三迁故事就是体现了周围人对你成长的影响,人在交友的过程中不断学习,潜移默化地受到对方的影响,这种影响也会伴随着人的一生,正所谓:“近朱者赤,近墨者黑。”
用机器学习的话说,距离你最近的K个“邻居”,决定了你是什么样的人,距离你最“近”的那些朋友,能看出你最真实的生活状况。
- 人有不同的气质,你是什么样的气质,就会吸引什么样的人。你若优秀,自然会吸引优秀的人,从一个人朋友的身上,往往也能找到这个人的影子。
你在受到周围人的影响同时,也会影响其他人;类似“环境影响生物,生物改造环境”、“实践决定认知,而认知对实践有反作用”,都是说明作用是相互的。
围棋盘上的每一颗棋子无论身处何处都会影响整盘棋的胜负,只不过随着距离的增大,影响力会快速衰减,但永远不会为零。
本身处于周围人影响的同时,我们也可以通过改变自身而影响他人。舍友喊你打游戏时,叫上他一起自习;朋友约你去网吧,劝他来图书馆。
- 眼界的高低,决定你格局的大小。
当你把K取成1时,你的眼睛只看到手上的事物就停下了,心甘情愿接受周围的同化;你把脚步局限在屋子里,舒舒服服地躺在沙发上摆烂;你只关心当下,得过且过是一天,一言不合就摆烂…
但是如果你尝试把K取成100呢?你可以通过网络了解到时代当今热点,国家出台新政策,政府发布新规范,行业迎来新机遇,就业有了新方向…看似距离我们很远的事物其实都和我们生活息息相关;
十鸟在林,不如一鸟在手,眼界高不仅看到当下,更要看到未来;格局大不计较眼前的小小得失,而是关注整盘棋的胜负。
更多推荐
所有评论(0)